Servicios Personalizados
Revista
Articulo
Links relacionados
Compartir
Ciencias Psicológicas
versión impresa ISSN 1688-4094versión On-line ISSN 1688-4221
Cienc. Psicol. vol.18 no.1 Montevideo 2024 Epub 01-Jun-2024
https://doi.org/10.22235/cp.v18i1.3286
Original Articles
Validation of a model of positive and negative personality traits as predictors of psychological well-being using machine learning algorithms
1 Universidad de Buenos Aires; Universidad de Palermo; Conicet, Argentina
2 Universidad de Buenos Aires; Universidad de Palermo; Conicet, Argentina, mllupano2@gmail.com
3 Universidad de Buenos Aires, Argentina
4 Universidad de Buenos Aires; Universidad de Palermo, Argentina
The objective of the study was to verify a predictive model of positive and negative personality traits taking psychological well-being as a criterion through the implementation of machine learning algorithms. 2038 adult subjects (51.9 % women) participated. For data collection were used: Big Five Inventory and Mental Health Continuum-Short Form. In addition, to assess the positive and negative personality traits, the already validated items of the positive (HFM) and negative trait models (BAM), were used jointly. Based on the findings found, it was possible to verify that the predictive efficacy of the tested model of positive and negative traits, derived from a lexical approach, was superior to the predictive capacity of normal personality traits for the prediction of well-being.
Keywords: positive traits; negative traits; personality; psychological well-being; algorithms
El objetivo de este estudio fue verificar un modelo predictivo de rasgos de personalidad positivos y negativos tomando como criterio el bienestar psicológico mediante la implementación de algoritmos de machine learning. Participaron 2038 sujetos adultos (51.9 % mujeres). Para la recolección de datos se utilizó: Big Five Inventory y Mental Health Continuum-Short Form. Además, para evaluar los rasgos positivos y negativos de personalidad se utilizaron los ítems ya validados de los modelos de rasgos positivos (HFM) y negativos (BAM) de forma conjunta. A partir de los hallazgos encontrados se pudo verificar que la eficacia predictiva del modelo testeado de rasgos positivos y negativos derivados de un enfoque léxico resultó superior a la capacidad predictiva de los rasgos normales de personalidad para la predicción del bienestar.
Palabras clave: rasgos positivos; rasgos negativos; personalidad; bienestar psicológico; algoritmos
O objetivo do estudo foi verificar um modelo preditivo de traços de personalidade positivos e negativos tendo como critério o bem-estar psicológico por meio da implementação de algoritmos de machine learning. Participaram 2.038 sujeitos adultos (51,9 % mulheres). Para a coleta de dados foram utilizados: Big Five Inventory e Mental Health Continuum-Short Form. Além disso, para avaliar os traços de personalidade positivos e negativos, foram utilizados conjuntamente os itens já validados dos modelos de traços positivos (HFM) e negativos (BAM). Foi possível verificar que a eficácia preditiva do modelo testado de traços positivos e negativos derivados de uma abordagem lexical foi superior à capacidade preditiva de traços normais de personalidade para a predição do bem-estar.
Palavras-chave: traços positivos; traços negativos; personalidade; bem-estar psicológico; algoritmos
Since the 20th century, the positive and negative characteristics of people have become the object of interest in psychology (McCullough & Snyder, 2000; Peterson & Seligman, 2004). Historically, these issues have been addressed from different approaches. Empirical approaches, which relate to the study of human characteristics, can be classified in two ways: theory-driven and data-driven. Theory-driven approaches design a priori a theoretical model, which is then corroborated with empirical data. Data-driven approaches are characterized as studies in which empirical data are analyzed with the aim of identifying clusters of elements (e.g., positive characteristics) in order to find a generalization of them, seeking to achieve their replication in different populations (Chow, 2002).
Regarding positive characteristics, there are several models that have explored and studied this topic. Peterson and Seligman's (2004) classification of virtues and strengths falls into the category of theory-driven approaches. The authors proposed a list of 24 character strengths, corresponding to six virtues (Courage, Justice, Humanity, Wisdom, Temperance and Transcendence). More recently, Kaufman et al. (2019) proposed a positive trait model called Light Triad (as opposed to the Dark Triad model -Paulhus & Williams, 2002- which will be further discussed) that includes three traits: Kantianism (traits oriented to treat others as ends in themselves and not as means to an end); Humanism (traits oriented to respect the dignity and value of each individual); Faith in humanity (traits oriented to believe and trust in the goodness of others).
In terms of data-driven approaches, several examples can be cited. On the one hand, Walker and Pitts (1998) sought to analyze conceptions of moral excellence by asking participants to identify attributes that a highly moral person possesses. With these data, they generated a set of moral descriptors. Six clusters of attributes were found: principled idealist, trustworthy and loyal, upright, caring, fair, and finally, confident. Cawley et al. (2000) identified 140 dictionary words that corresponded to moral virtues. With these adjectives and nouns and through factor analysis procedures, the authors found four dimensions of positive virtues: empathetic, orderly, resourceful, and serenity. De Raad and van Oudenhoven (2011), using a psycholexical approach, asked students and psychologists to identify words describing virtues from a series of dictionary terms. From this study emerged a six-factor model of virtues consisting of sociability, achievement, respect, vigor, altruism, and prudence. Another study from the data-driven perspective was conducted by Morales-Vives et al. (2014). The authors identified 209 virtue descriptors. As a result of this procedure, a model of seven dimensions was proposed: self-confidence, reflection, serenity, rectitude, perseverance and effort, compassion, and sociability.
More recently, Cosentino and Castro Solano (2017) explored human positive characteristics, using a psycholexical approach. This type of approach was first employed by Allport and Odbert (1936) and gives a fundamental place to the lexicon, as a basis upon which a classification of human differences can be built (De la Iglesia & Castro Solano, 2020). In a first study, Cosentino y Castro Solano (2017) identified positive characteristics from the point of view of common individuals, with the aim of developing a model of human positive trait factors, socially shared, and that could be replicable in other populations. This research was innovative because previous studies focused only on characteristics associated with moral traits, while talents and abilities had been systematically excluded. This model included these characteristics more broadly, encompassing those related to performance (such as intelligence).
That research resulted in the High Five Model (HFM; Cosentino & Castro Solano, 2017). Five traits were found to be present, to vary in each individual, to be measurable and, moreover, to be increased or reduced by internal and/or external influences. According to the authors, the five HFM factors are: Erudition (a trait associated with thinking solutions, having a desire to learn), Peace (associated with thinking calmly and trusting that things will take their natural course), Joviality (expressed in desires to make others laugh, have fun and amuse others), Honesty (associated with being transparent and having a tendency to tell the truth) and Tenacity (expressed in pursuing goals and seeking to accomplish objectives with effort).
On the other hand, psychology has also been interested in the negative characteristics of human personality. Studies proposing negative trait models have generally used a theory-driven approach. One model that generated great interest is the Dark Triad of Personality (Paulhus & Williams, 2002), which proposes three negatives, but not pathological, personality traits (Machiavellianism, Narcissism and Psychopathy). This model was expanded after its creation to include a new dimension: Sadism (Jones & Paulhus, 2014).
Cosentino y Castro Solano (2023) proposed a model of negative characteristics from the perspective of common individuals, as well as the model of positive traits. Using the psycholexical approach, they found three factors that showed a good data fit. They inductively named this derived model Brutalism, Arrogance, and Malignism (BAM). The three factors are: Brutalism (careless, unstable, rude, unbearable individuals), Arrogance (arrogant, conceited, boastful and pedantic subjects) and Malignism (people who cheat, perform immoral acts, mean, spiteful, etc.). This model is more recent than the model of positive personality traits and is in its validation phase.
Well-being and positive and negative personality models
The study of the link between personality and well-being has been widely studied since personality traits are considered the best known predictors of subjective experiences (Tkach & Lyubomirsky, 2006). Classical research that attempted to explain well-being from personality variables mostly considered normal personality traits (Anglim et al, 2020), mainly from the Five Factor Model (FFM) (Costa & McCrae, 1984). However, in recent years, attention has begun to be paid to the relationship with negative (Blasco-Belled et al., 2024) and positive personality traits (Kaufman et al., 2019).
In the present research well-being was approached from the perspective of Keyes (2002, 2005) since it is one of the most widely used in international studies. The author understands mental health as a continuum called languishing-flourishing, in which individuals can be classified into three groups: languishing, made up of subjects who present difficulties in life, lack of commitment, feeling of emptiness; flourishing, subjects who have an optimal development in their lives; and, finally, moderate mental health, which is made up of subjects who do not fall into the two previous classifications, presenting a moderate level. This model, then, understands health and illness as a continuum, through the presence or absence, in addition to the degree of hedonic (emotional) and eudaemonic (social and psychological well-being) well-being.
Regarding the relationship between well-being and FFM traits, a recent meta-analysis (Anglim et al., 2020) reports that the traits that show the strongest association with both hedonic and eudaemonic well-being are Neuroticism, Extraversion and Conscientiousness.
If the positive personality traits are consider, some studies show that Peterson and Seligman's character strengths model (Peterson & Seligman, 2004; Park et al., 2004) allows predicting life satisfaction, with the strengths of hope, gratitude, curiosity and love being the most associated. Likewise, taking the most recent model of Kaufman et al. (2019), it was found that the three light traits correlate positively with life satisfaction and global well-being (Kaufman et al., 2019; Stavraki et al., 2023).
In relation to the positive trait model analyzed in the present research, the High Five model, previous studies demonstrated that these positive personality factors had incremental validity in predicting psychological well-being (hedonic and eudamonic) over the FFM model (Cosentino & Castro Solano, 2017). In addition, another study showed that these factors were negatively associated with indicators of psychological symptomatology, low risk for medical illness, and pathological personality traits (Castro Solano & Cosentino, 2017). Particularly the traits Peace and Joviality were the traits most strongly associated with low risk of contracting both medical and psychological illnesses. In another study conducted with university students, it was verified that high factors, besides promoting psychological well-being, allowed predicting both adaptation to university life and academic performance of students (Castro Solano & Cosentino, 2019). In this case, Tenacity and Erudition factors were the two positive personality factors most strongly associated with the perception of adjustment to university life.
Regarding antecedents that relate well-being with negative personality traits, studies carried out with the Dark Triad model show that Machiavellianism and Psychopathy are the traits that best predict well-being in a negative way, especially eudaemonic traits (Liu et al., 2021). A recent meta-analysis that included the analysis of 55 studies that worked with negative personality models reported that Antagonism, Disinhibition and Machiavellianism were related to lower levels of well-being. They also showed that age and gender moderated some of these associations (Blasco-Belled et al., 2024).
If the negative trait model analyzed in the present investigation is considered, the BAM model, previous results showed negative associations with life satisfaction and positive associations with psychopathological symptoms (Cosentino & Castro Solano, 2023).
The present research
The purpose of this research is to study a model of positive and negative traits derived from a psycholexical approach for the prediction of both hedonic and eudaemonic psychological well-being. In relation to positive traits the High Five model was considered (Cosentino & Castro Solano, 2017). In relation to negative personality traits, a model of psycholexic characteristics specially designed for local population was taken into account (Cosentino & Castro Solano, 2023).
According to the above, the reasons for choosing these models lie, on the one hand, in the fact that modern personality models (e.g., positive and negative traits) add additional variance for the explanation of psychological well-being over classical personality models (Cosentino & Castro Solano, 2017; Castro Solano & Cosentino, 2019). On the other hand, both models have been developed locally, and from a psycholexical approach, which decreases the risk of falling into an ethic-imposed perspective given that the cultural equivalence of some psychological constructs (e.g., positive traits) is a matter of constant debate (Lopez et al., 2002).
A novelty of this study refers to the use of machine learning algorithms. For some years now these algorithms have become popular in psychology especially for predictive purposes (Yarkoni & Westfall, 2017) as they allow discovering patterns and developing predictive models in a variety of circumstances and application fields of psychology such as psychometrics, experimental psychology, diagnosis, treatment, monitoring and personalized patient care (Dey, 2016; Dhall et al., 2020; Dwyer et al, 2018; Jacobucci & Grimm, 2020; Koul et al, 2018; Lin et al, 2020; Orrù et al, 2020; Shatte et al, 2019). For example, some research using machine learning algorithms allowed identifying personality traits through social media posts (Bleidorn & Hopwood, 2019; Park et al., 2015), or preferred music based on Facebook Likes (Nave et al., 2018). In the clinical setting a recent study, through machine learning algorithms, was able to identify specific predictors of the coping strategies of a large group of patients undergoing cognitive behavioral treatment (Gómez Penedo et al., 2022).
Therefore, the contributions of this research fall, on the one hand, on the theoretical level. Classical research that explained well-being from personality variables considered mostly normal personality traits from the FFM (Anglim et al., 2020). In this case, the main contribution of this study is to present a model that includes other personality variables not commonly analyzed and that have become relevant in the field of personality study: negative traits and positive traits. Using models that consider new personality variables allows to increase the prediction of well-being (greater variance explained compared to traditional models). In addition, it is proposed to test the integrated functioning of positive and negative traits in the same model.
On the other hand, from the methodological point of view, the contribution lies in the inclusion of machine learning algorithms for the explanation of the criterion variables (psychological, emotional and social well-being). The classificatory algorithms help to improve, from a methodological point of view, the prediction by identifying subgroups of specific subjects. For their part, predictive algorithms allow: a) running the analyses with fewer statistical assumptions (e.g. normality, homogeneity of variance), b) considering a large number of variables without affecting the results (high dimensionality), c) estimating the generalizability of the results through cross-validation techniques, a very robust technique in which the data are divided into training and test phases (Dwyer et al, 2018). This last procedure and the incorporation of regularization techniques (hyperparameters in Lasso and Ridge regressions) are far superior to the use of classical linear regressions as they allow improving the accuracy and generalizability of the results (Delgadillo et al., 2020; Zou & Hastie, 2005).
Based on the above, the objectives of this study are: (1) To verify a predictive model of positive and negative personality traits taking into account psychological well-being (personal, emotional and social) as a criterion by implementing machine learning algorithms (Lasso, Ridge and Random Forest regularization); (2) To verify the predictive validity of the personality model of positive and negative traits over the model of normal personality traits, taking psychological well-being (personal, emotional and social) as a criterion by implementing machine learning algorithms (Lasso, Ridge and Random Forest regularization); (3) Verify the accuracy, sensitivity and specificity of the predictive model of positive and negative personality traits to identify people with high and low psychological well-being using machine learning algorithms (support vector machine).
Method
Participants
Participants were volunteers and answered anonymously. The inclusion criterion was that they should be Argentine citizens (18 years of age or older). The final sample consisted of a total of 2038 Argentine participants. The 51.9 % (n = 1058) were women and 48.1 % (n = 980) were men. The mean age was 40.4 (SD = 14.7), with ages ranging from 18 to 91 years. Regarding marital status, 33.2 % (n= 676) were single, 16.5 % (n = 336) were dating, 26.1 % (n = 531) were married, 20.4 % (n = 416) reported being divorced or separated, while 3.9 % (n = 79) were widowed. Regarding their occupations, 53.4 % (n = 1088) were employed, 18.3 % (n = 374) were not actively working at the time of the survey, 20.1 % (n = 409) reported being self-employed, 0.5 % (n = 11) were unpaid workers, 2.6 % (n = 54) were homemakers, while 5 % (n = 102) were employers. Finally, in relation to socioeconomic level, 2.0 % (n= 40) belonged to a low level, 14.3 % (n = 292) belonged to a medium-low level, 63.5 % (n= 1294) to a medium level, 17.8 % (n = 363) declared belonging to a medium-high level, and 2.4 % (n= 49) of the sample belonged to a high socioeconomic level.
Materials
Big Five Inventory (BFI; John et al., 1991; Argentine adaptation Castro Solano & Casullo, 2001). It is a 44-item instrument that assesses the Big Five personality traits (Extraversion, Agreeableness, Conscientiousness, Neuroticism, and Openness to experience). The author of the test demonstrated its validity and reliability in general adult North American population groups. These studies verified the concurrent validity with other recognized instruments that assess personality. Studies carried out in Argentina verified the factorial validity of the instrument for adolescent population, non-consultant adult population and military population (Castro Solano & Casullo, 2001). In all cases, a five-factor model was obtained that explained about 50 % of scores variance. Adequate internal consistency values (ordinal alpha) were obtained for this sample: extraversion = .76; agreeableness = .79; conscientiousness= .82; neuroticism = .74; openness to experience = .69.
Mental Health Continuum-Short Form (MHC-SF; Keyes, 2005; Argentine adaptation Lupano Perugini et al., 2017). This 14-item instrument assesses the degree of: a) Emotional well-being, in terms of positive affect and life satisfaction (hedonic well-being), b) Social well-being (including the facets of acceptance, actualization, social contribution, coherence and social integration), c) Personal well-being in terms of Ryff's (1989) theory (autonomy, control, personal growth, personal relationships, self-acceptance and purpose). The MHC-SF has shown good evidence of internal consistency (> .70) and discriminant validity in samples of adults from various countries. The three-factor structure of the test (emotional, personal and social) has been verified in these studies. Validation studies of this instrument in Argentina have confirmed the factor structure of the instrument and have given evidence of good convergent validity and internal consistency in adult population (Lupano Perugini et al., 2017). The reliability of the subdimensions for this sample were (ordinal alpha): emotional well-being = .80; social well-being = .74; personal well-being = .77.
Positive and negative personality traits
To assess positive and negative personality traits from a psycholexical point of view, the already validated items from High Five Model (HFM; Cosentino & Castro Solano, 2017) and those derived from the negative trait model (BAM; Cosentino & Castro Solano, 2023) were used:
High Five Model (positive traits) (Cosentino & Castro Solano, 2017). To assess the positive personality traits, the HFM model was taken into account. The scale is composed of 23 items that assess the five high factors Erudition, Peace, Joviality, Honesty and Tenacity. These factors were obtained through an inductive procedure based on the point of view of common individuals on positive human characteristics. People are asked to respond to each item, for example, "I have patience" on a Likert scale ranging from 1(never) to 7 (always). The higher the score on each subscale, the higher in the factor. Studies on the HFI demonstrated convergent and divergent validity in relation to the Values in Action classification of Peterson and Seligman (2004) and incremental validity over the factors and facets of the Big Five personality model for the prediction of psychological, emotional and social well-being. Adequate internal consistency values (ordinal alpha) were obtained for this sample: erudition = .84, peace = .86, joviality = .88, honesty = .88, and tenacity = .86.
BAM Model (negative traits) (Cosentino & Castro Solano, 2023). To assess the negative personality traits, the negative trait model was taken into account through the items of the BAM inventory (Brutalism, Arrogance, Malignism). The BAM model is a recently created model that is composed of three factors obtained inductively to evaluate negative personality traits. The inductive procedure followed a psycholexical approach through a corpus of words obtained from ordinary people who reported characteristics considered "negative" and which were socially shared. The brutalism factor is defined by a set of negative individual traits such as being unbearable, careless, unstable, ridiculous; the arrogance factor is defined by a set of negative characteristics such as being arrogant, conceited, arrogant and narcissistic; and the malignism factor is defined by characteristics such as being corrupt, deceitful, immoral, malicious, and despicable, among others. Participants are assessed by asking them to respond to the item, for example, "I am conceited" on a Likert scale ranging from 1 (never) to 7 (always). In a preliminary study (Cosentino & Castro Solano, 2023) the model demonstrated good construct validity and positive associations with psychopathological symptoms and negative associations with life satisfaction. The 22 items of the model are grouped into 3 factors that were confirmed by confirmatory factor analysis techniques that demonstrated a good fit of the model to the data (Robust Weighted Least Squares Estimator (DWLS, robust) χ2 (206) = 323.00, p < .05, fit estimators CFI = .986, SRMR = .060, and RMSEA = .041 (90 % Confidence Interval = .032 - .049). It was decided to include this personality model as a complement to the positive trait model (HFI) in order to further study its predictive validity. Adequate internal consistency values (ordinal alpha) were obtained for this sample: brutalism = .85, arrogance = .91 and malignism = .85.
Procedure
Data were collected by students who were doing a research internship at a private university in the city of Buenos Aires, Argentina. The participants were volunteers and did not receive any compensation for their collaboration. Data were collected during 2021 and 2022. The materials were administered on-line using the SurveyMonkey application. The survey home page requested the participant's consent, assured the anonymity of the data and its exclusive use for research purposes. Data collection was supervised by one of the authors of this article. The research followed international ethical guidelines (APA and NC3R) and those of the National Council for Scientific and Technical Research (Conicet) for ethical behavior in the Social Sciences and Humanities (Resolution No. 2857, 2006) and has the approval of the corresponding ethics committees.
For data analysis, the statistical package Jamovi (2022) was used through the R environment, Version 4.1.2 (R Core Team, 2021) for correlation and logistic regression calculations. For the implementation of machine learning algorithms, the R software (R Core Team, 2021) was used. The packages caret, glmnet, randomForest and e107 were used.
Data Analysis
Different machine learning algorithms were used to validate a model of positive and negative personality traits for the prediction of different types of psychological well-being (research objectives 1 and 2), taking the normal personality trait model as a starting point.
As a first step, the participants were randomly divided into two subsets. The first one was called the training data set (2/3 of the participants, n = 1528), the second subset was called the validation data set (1/3 of the sample, n = 510). All continuous variables were transformed to z-scores.
Different regularization techniques (Lasso regression and Ridge regression) were used to verify the predictive validity of two personality models (normal personality traits vs. negative and positive traits) on psychological well-being. For comparison purposes, a linear regression (ordinary least squares) was carried out in which all predictors of both models (personality variables) were included. The regularization techniques employed are extensions of ordinary least squares regression and include different types of penalties in the regression coefficients with the purpose of improving the interpretation of the models (Zou & Hastie, 2005). Ridge regression is used when there is multicollinearity among the predictors. The advantage of this type of regression is to keep all predictors in the model and the disadvantage is that it does not produce parsimonious models for the reason mentioned above (Delgadillo et al., 2020; Friedman et al., 2010). Lasso regularization is used in cases where there are several predictors with coefficients close to zero and some with larger coefficients. This type of regression automatically selects the predictors relevant to the model, discards the others, and produces more parsimonious and interpretable models (Zou & Hastie, 2005). The Random Forest algorithm was also used, which is a nonparametric recursive partitioning technique that combines different decision trees in such a way that a random vector depends on each tree and is tested independently. The advantage of this methodology is that it randomly incorporates predictors considered weak, generally ignored in other models, due to the dominance of predictors considered stronger (Garge et al., 2013). Also, this methodology allows the inclusion of a larger number of predictors. It differs from the Lasso and Ridge regularization as it allows working with complex associations and interactions and nonlinear relationships. Lasso and Ridge have two parameters λ (Lambda) and α (alpha). α is arbitrarily set to 1 for Lasso regression and 0 for Ridge. The parameter λ was calculated using the cross-validation technique. The glmnet package (Friedman, et al., 2010) was used to set it.
In the Random Forest algorithm, the parameter to be estimated is the mtry, which is defined by the number of predictors that are randomly assigned to each node. The parameter tuning was performed using the randomForest package (Liaw & Wiener, 2002), through successive iterations, for the selected predictor models.
Next, the models were trained using the training data set. The models were then compared in terms of RMSE (root mean squared error) and R 2 in order to select the model with the best goodness of fit. Once this was done, the model was tested on the validation data set and the results obtained on both data sets were compared. Figure 1 summarizes the steps carried out in the data analysis.
A similar procedure was followed for objective 3. In this case, the Support Vector Machine (SVM) algorithm was used. The SVM is a very robust supervised machine learning algorithm that allows data analysis for both classification and regression purposes (Gareth et al., 2013). Given a set of training data in which a series of predictors are included, being the criterion to be predicted a binary classification, the algorithm allows to establish predictions that are tested on a new sample (validation data set or test set). The algorithm has the task of establishing a hyperplane that separates in the best possible way both binary classes (criteria to be predicted, in this research high and low psychological well-being). The evaluation of the model is done by the ability of the algorithm to be able to predict more accurately the membership of each class. The SVM has 4 parameters (Kernel, Regularization - C -, Gamma and Epsilon) that in this case were estimated using the e1071 package. For comparative purposes, a logistic regression was estimated in which the predictors of both personality models were included as well as the criterion of belonging to the high and low well-being group. The indicators of precision, sensitivity and specificity obtained by logistic regression and by the SVM were then compared. The purpose of this procedure was to establish the predictive capacity of both personality models on psychological well-being.
Results
Predictive Models of Well-Being (Lasso, Ridge and Random Forest algorithms)
First, correlations were calculated between the predictor variables of both models (normal, positive and negative personality traits) and the three types of well-being (emotional, personal, social and total) in the training data set. All correlations obtained between the predictor variables (personality traits) and the criterion variables (well-being types) were significant p < .0001.
Second, the tuned parameters of the three predictive algorithms (Lasso Regression, Ridge and Random Forest) of psychological well-being as a function of normal personality traits and positive and negative personality traits were calculated. Both the parameter λ of the regression models and the mtry of the Random Forest algorithm were tuned using the cross-validation technique.2
Third, the results of the different models are presented, including the linear least squares regression in terms of RMSE (root mean squared error) and R 2 (Tables 1 2 3 4). The model with the highest explained variance (highest R 2 ) and lowest RMSE is considered as the model selection criterion. The results show that the best predictive model corresponds to the Random Forest algorithm. In the training data set, both for the model of normal personality traits (base model) and for the model of positive and negative traits (model to be tested in this research), the Random Forest algorithm presented the lowest RMSE both for the prediction of total well-being and for emotional, personal and social well-being.
In relation to the variance explained, the model of normal personality traits (base model) explained 32 % of the variance of total well-being, 34 % of personal well-being, 23 % of emotional well-being and 11 % of social well-being. The model of positive and negative traits (model to be tested in this research) reached similar values and in some cases somewhat higher, explaining 34 % of the variance of total well-being, 36 % of personal well-being, 24 % of emotional well-being and 16 % of social well-being.
These results were validated on another dataset (test set) reaching similar values (cross-validation). In this validation subsample, the Random Forest algorithm presented the lowest RMSE for the prediction of total well-being, as well as for emotional, personal and social well-being. Therefore, this algorithm had the best performance to explain the model to be tested in this research (Table 5). To explore the contribution of each specific personality dimension (trait) within the Random Forest technique, the decreasing mean Gini (i.e., IncNodePurity (INP)) was calculated for the positive and negative trait model. This index shows that, for the prediction of total well-being, the most important predictors turned out to be Erudition (INP = 48.48) and Tenacity (INP = 53.68). The results are similar for personal well-being: Tenacity (INP = 57.40) and Erudition (INP = 56.11). For emotional well-being they were Tenacity (INP = 65.66), Peace (INP = 52.34) and Malignism (INP = 51.77) and for social well-being, Erudition (INP = 49.59) and Peace (INP = 49.38).
Predictive Models of High and Low Psychological Well-Being (Support Vector Machine Algorithm)
The Support Vector Machine (SVM) algorithm was used to predict psychological well-being taking as predictors the normal personality models and the positive and negative trait model.
First, two logistic regressions were calculated for both models taking normal personality traits and positive and negative personality traits as predictor variables. In both cases, high/low well-being was considered as a criterion. To form the latter variable, the total well-being variable was converted into a dummy variable (0 = low well-being; 1= high well-being) taking into account the mean (M = 3.05, SD = .86) in the total well-being score for each participant, above the mean was considered high well-being and below the mean was considered low well-being.
The normal personality traits model (base model) was statistically significant, χ2(5,N= 1528) = 431.3.3, p < .001, this indicates that the five predictors composing the model together allow distinguishing between people with high and low psychological well-being. The variance explained was 24.6 % (Cox and Snell's R2). The accuracy of the model (percentage of correct positive predictions) for identifying cases of high well-being was 72 %. Sensitivity (percentage of high well-being cases detected) was 74 % and specificity (percentage of low well-being cases detected) was 69 %. All predictors in the model (personality traits) were significant (p < .001), extraversion and neuroticism recorded the highest values.
The model of positive and negative traits (model to be tested in this research) was statistically significant, χ2 (8, N = 1528) = 414, p < .001, this indicates that the seven predictors that compose the model as a whole allow distinguishing between people with high and low psychological well-being. The variance explained was 24 % (Cox and Snell's R2). The accuracy of the model in identifying cases of high well-being was 71 %. Sensitivity (percentage of high well-being cases detected) was 75 % and specificity (percentage of low well-being cases detected) was 67 %. All model predictors (personality traits) were significant (p < .001), except for two of the negative personality traits (brutalism and arrogance).
The accuracy of both models (base model vs. model to be tested in this research) in predicting high/low psychological well-being was similar, with the normal personality trait model having the best goodness of fit (AIC 1696 vs. 1719).
Second, the SVM algorithm was used to establish the prediction efficiency of both personality models on high/low psychological well-being. The parameters used were SVM of the regression type, Radial Kernel, gamma = .125 and epsilon = .01. The support vectors were 138. The SVM data indicate that the accuracy of both personality models is similar, as well as the indicators of sensitivity and specificity. Sensitivity (percentage of high well-being cases detected) was 75 % and specificity (percentage of low well-being cases detected) was 61 % for both the normal personality trait model and the positive and negative trait model.
Table 1: Fit of Models for Predicting Total Well-Being, according to Positive and Negative Normal Personality Traits (training set: n= 1528 and test set: n= 510)
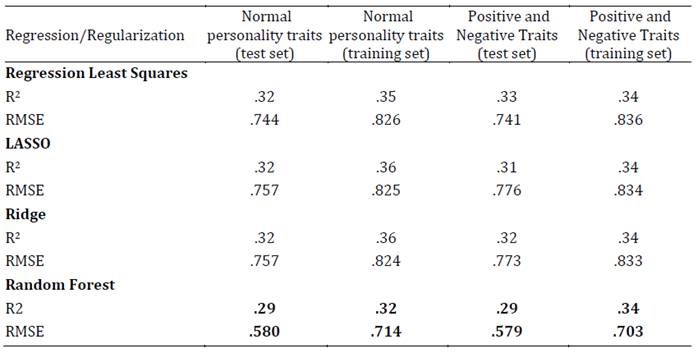
Table 2: Fit of Models for Predicting Personal Well-being, according to Positive and Negative Normal Personality Traits (training set: n= 1528 and test set: n= 510)
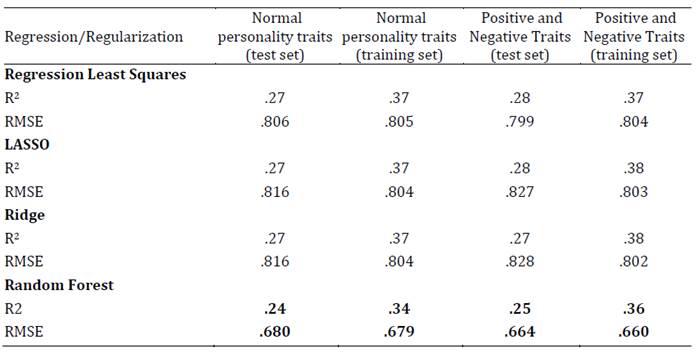
Table 3: Fit of Models for Predicting Emotional Well-being, according to Positive and Negative Normal Personality Traits (training set: n= 1528 and test set: n= 510)
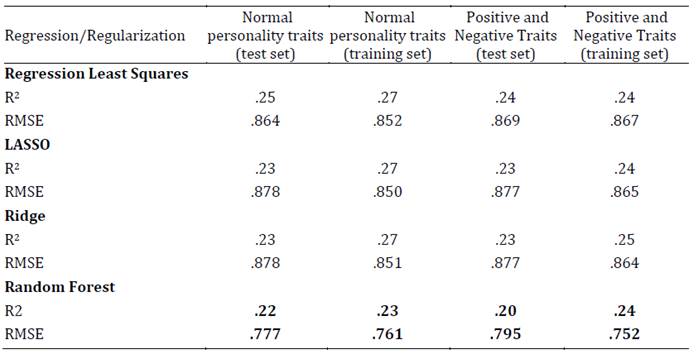
Table 4: Fit of Models for Predicting Social Well-being, according to Positive and Negative Normal Personality Traits (training set: n= 1528 and test set: n= 510)
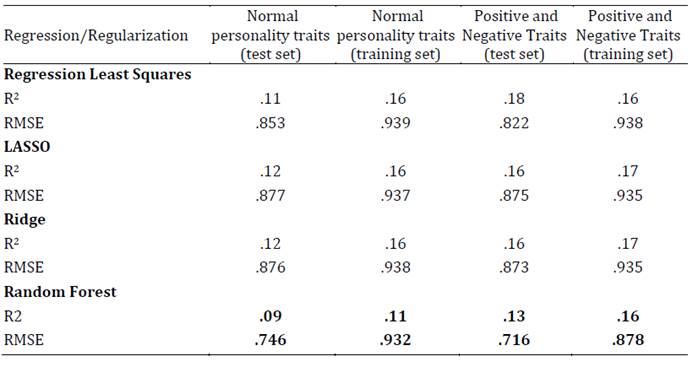
Discussion
The main goal of this study was to verify an integrated predictive model of positive and negative personality traits based on psychological well-being (personal, emotional and social) by implementing machine learning algorithms.
Based on three predictive algorithms (Lasso Regression, Ridge and Random Forest) the predictive validity of the tested model of positive and negative traits, derived from a lexical model, was tested, which was similar and even somewhat superior to the predictive validity of the FFM for the prediction of both hedonic and eudaemonic well-being.
In relation to the predictive validity of each positive and negative traits included in the integrated model, it was observed that all were significant predictors with the exception of two of the negative personality traits (Brutalism and Arrogance). As expected, the positive traits were all significant predictors as shown by previous studies conducted with the High Fives Model (HFM; Castro Solano & Cosentino, 2017; Castro Solano & Cosentino, 2019; Cosentino & Castro Solano, 2017). The traits that had the most weight in the prediction of well-being (total and personal) were Erudition and Tenacity while Peace and Malignism added for the prediction of emotional and personal well-being. Overall, the positive traits discussed (Erudition and Tenacity) had a protective effect on psychological well-being. This result also confirms previous findings with this same model of positive traits, when predicting academic adjustment to university life. (Castro Solano & Cosentino, 2019). Malignism (e.g., corrupt, deceitful, malicious) is the negative trait most conceptually linked to its equivalent Machiavellianism and Psychopathy of the Dark Triad. This result is consistent with previous studies in which these negative traits are those that best predict negative associations with well-being, especially of a eudaemonic nature (e.g., Blasco-Belled et al., 2024; Liu at al., 2021). Finally, Peace, which would be the opposite of neuroticism in the FFM is the trait most associated with emotional and social well-being. These findings are in line with previous studies discussed on the relationship between personality traits and well-being.
On the other hand, the Support Vector Machine (SVM) algorithm was used to verify the accuracy, sensitivity and specificity of the predictive model of positive and negative personality traits to identify people with high and low psychological well-being. The results found show that for both the base model (normal traits) and the tested model (integrated positive and negative traits), the accuracy, sensitivity and specificity were similar. It should be noted that, according to what was observed, it can be inferred that the specificity, that is, the ability of these models to detect cases of low well-being is lower with respect to their sensitivity and accuracy. This may be influenced by the lower predictive capacity shown by the traits of the negative trait model (BAM) -which tend to be more associated with low well-being-, with respect to the positive trait model (HFM) -more associated with high well-being-. For this reason, future studies should continue to study the content validity of the BAM inventory in order to identify whether the items are representative of the construct assessed.
The strength of the study lies in two central aspects. On the one hand, an integrated model of positive and negative traits for the prediction of well-being is verified. In addition, this model has been developed from a psycholexical approach that allows us to capture the local (emic) and singular variants of the constructs studied in each particular culture. This aspect is not minor given the debate that has been generated, mainly regarding the universalization of some positive constructs such as the virtues and strengths model of Peterson and Seligman (2004) (Lopez et al., 2002). Many researchers have warned about the possible ethnocentric biases that can be carried out by dealing with classifications from an ethic-imposed perspective (Christopher & Hickinbotton, 2008; Snyder et al., 2011). Hence the need to generate local models for the evaluation of this type of phenomena that may be influenced by cultural aspects. The advantage of this study, over those already carried out locally, is that it shows the predictive power of these traits in an integrated model. Although the antecedents on other models of positive and negative traits show that they are not opposing traits or that they can be understood as a continuum (Kaufman et al., 2019), it is interesting to verify their predictive power jointly.
Another of the strengths of the present study lies in the use of a novel methodology based on machine learning algorithms for predictive purposes (Yarkoni & Westfall, 2017), which provides objectivity and rigor to the research developed. It is also noteworthy the large number of participants in the sample that allowed to perform cross-validation techniques to select and estimate the best possible parameters of the models.
In terms of limitations, it should be mentioned that self-report measures were used for data collection, which may be influenced by a tendency to respond in a socially desirable way and affect the validity of the data and the inferences made. Another limitation of the work is the selection of machine learning algorithms, being limited to algorithms based on regression and recursive partitioning techniques (Random Forest). Current methodologies in the field of artificial intelligence even allow the use of very sophisticated algorithms for predictive purposes, which were not included in the present study.
The inclusion of both the positive and negative trait model based on a psycholexical approach, as well as the use of machine learning techniques, offer a very promising overview for the treatment of personality variables as predictors of a wide variety of psychological outcomes. The instruments derived from this model are composed of adjectives that people frequently use to describe themselves and others, have ecological and empirical validity, and allow the assessment of important psychological characteristics in a few minutes.
In future research it will be interesting to include these predictors to assess important psychological outcomes in specific applied domains, such as work (e.g. predicting job satisfaction, commitment and flow at work), academia (e.g. satisfaction with vocational choice) or even the field of psychological treatments (e.g. satisfaction with psychotherapy, efficacy of treatments).
REFERENCES
Allport, G. W. & Odbert, H. S. (1936). Trait-names: A psycholexical study. Psychological Monographs, 47(1), i-171. https://doi.org/10.1037/h0093360 [ Links ]
Anglim, J., Horwood, S., Smillie, L. D., Marrero, R. J., & Wood, J. K. (2020). Predicting psychological and subjective well-being from personality: A meta-analysis. Psychological Bulletin, 146(4), 279-323. https://doi.org/10.1037/bul0000226 [ Links ]
Blasco-Belled, A., Tejada-Gallardo, C., Alsinet, C., & Rogoza, R. (2024). The links of subjective and psychological well-being with the Dark Triad traits: A meta-analysis. Journal of Personality, 92, 584-600. https://doi.org/10.1111/jopy.12853 [ Links ]
Bleidorn, W., & Hopwood, C. J. (2019). Using machine learning to advance personality assessment and theory. Personality and Social Psychology Review, 23(2), 190-203. https://doi.org/10.1177/1088868318772990 [ Links ]
Castro Solano, A., & Casullo, M. M. (2001). Rasgos de personalidad, bienestar psicológico y rendimiento académico en adolescentes argentinos. Interdisciplinaria 18, 65-85. [ Links ]
Castro Solano, A., & Cosentino, A. (2017). High Five Model: Los factores altos están asociados con bajo riesgo de enfermedades médicas, mentales y de personalidad. Psicodebate. Psicología, Cultura y Sociedad, 17(2), 69-82. https://doi.org/10.18682/pd.v17i2.712 [ Links ]
Castro Solano, A., & Cosentino, A. (2019). The High Five Model: Associations of the high factors with complete mental well-being and academic adjustment in university students. Europe’s Journal of Psychology, 15(4), 656-670. https://doi.org/10.5964/ejop.v15i4.1759 [ Links ]
Cawley, M. J., Martin, J. E., & Johnson, J. A. (2000). A virtues approach to personality. Personality and Individual Differences, 28(5), 997-1013. https://doi.org/10.1016/s0191-8869(99)00207-x [ Links ]
Chow, S. L. (2002). Methods in psychological research. Encyclopedia of Life Support Systems. [ Links ]
Christopher, J. C., & Hickinbottom, S. (2008). Positive psychology, ethnocentrism, and the disguised ideology of individualism. Theory & Psychology, 18(5), 563-589. https://doi.org/10.1177/0959354308093396 [ Links ]
Cosentino, A., & Castro Solano, A. (2017). The High Five: Associations of the five positive factors with the Big Five and well-being. Frontiers in Psychology, 8, e1250. https://doi.org/10.3389/fpsyg.2017.01250 [ Links ]
Cosentino, A., & Castro Solano, A. (2023, en prensa). An Inductively derived Model of Negative Personality Traits. Testing, Psychometrics, Methodology in Applied Psychology. [ Links ]
Costa, P. T., & McCrae, R. R. (1984). The NEO Personality Inventory Manual. Psychological Assessment Resources. https://doi.org/10.1007/springerreference_184625 [ Links ]
De la Iglesia, G. & Castro Solano A. (2020). Inventario de los Cinco Continuos de la Personalidad -ICCP-. Evaluación de Rasgos Positivos y Patológicos de la Personalidad. Paidós Informatizados. [ Links ]
De Raad, B., & Van Oudenhoven, J. P. (2011). A psycholexical Study of Virtues in the Dutch Language, and Relations between Virtues and Personality. European Journal of Personality, 25(1), 43-52. https://doi.org/10.1002/per.777 [ Links ]
Delgadillo, J., & Gonzalez Salas Duhne, P. (2020). Targeted prescription of cognitive-behavioral therapy versus person-centered counseling for depression using a machine learning approach. Journal of Consulting and Clinical Psychology, 88(1), 14-24. https://doi.org/10.1037/ccp0000476 [ Links ]
Dey, A. (2016). Machine Learning Algorithms: A review. International Journal of Computer Science and Information Technologies, 7(3), 1174-1179. [ Links ]
Dhall, D., Kaur, R., & Juneja, M. (2020). Machine Learning: A review of the algorithms and its applications. En P. Singh, A. Kar, Y. Singh, M. Kolekar, & S. Tanwar (Eds.), Proceedings of ICRIC 2019. Lecture Notes in Electrical Engineering (pp. 47-63). Springer. https://doi.org/10.1007/9 78-3-030-29407-6_5 [ Links ]
Dwyer, D. B., Falkai, P., & Koutsouleris, N. (2018). Machine Learning approaches for clinical psychology and psychiatry. Annual Review of Clinical Psychology, 14, 91-118. https://doi.org/10.1146/annurev-clinpsy-032816-045037 [ Links ]
Friedman, J., Hastie, T., & Tibshirani, R. (2010). Regularization paths for generalized linear models via coordinate descent. Journal of Statistical Software, 33(1), 1-22. https://doi.org/10.18637/jss.v033.i01 [ Links ]
Gareth, J., Daniela, W., Trevor, H., & Robert, T. (2013). An introduction to statistical learning: with applications in R. Spinger. https://doi.org/10.1111/j.1467-9868.2005.00503.x [ Links ]
Garge, N. R., Bobashev, G., & Eggleston, B. (2013). Random forest methodology for model-based recursive partitioning: the mobForest package for R. BMC bioinformatics, 14(1), 1-8. https://doi.org/10.1186/1471-2105-14-125 [ Links ]
Gómez Penedo, J. M., Schwartz, B., Giesemann, J., Rubel, J. A., Deisenhofer, A. K., & Lutz, W. (2022). For whom should psychotherapy focus on problem coping? A machine learning algorithm for treatment personalization. Psychotherapy Research, 32(2), 151-164. https://doi.org/10.1080/10503307.2021.1930242 [ Links ]
Jacobucci, R., & Grimm, K. J. (2020). Machine Learning and psychological research: the unexplored effect of measurement. Perspectives on Psychological Science: a Journal of the Association for Psychological Science, 15(3), 809-816. https://doi.org/10.1177/1745691620902467 [ Links ]
John, O. P., Donahue, E. M., & Kentle, R. L. (1991). Big Five Inventory (BFI) (Database record). APA PsycTests. https://doi.org/10.1037/t07550-000 [ Links ]
Jones, D. N., & Paulhus, D. L. (2014). Introducing the short dark triad (SD3) a brief measure of dark personality traits. Assessment, 21(1), 28-41. https://doi.org/10.1177/1073191113514105 [ Links ]
Kaufman S. B., Yaden D. B., Hyde, E., & Tsukayama, E. (2019). The light vs. dark triad of personality: contrasting two very different profiles of human nature. Frontiers in Psychology, 10, e467. https://doi.org/10.3389/fpsyg.2019.00467 [ Links ]
Keyes, C. L. M. (2002). The mental health continuum: From languishing to flourishing in life. Journal of Health and Social Behavior, 43, 207-222. https://doi.org/10.2307/3090197 [ Links ]
Keyes, C. L. M. (2005). The subjective well-being of America’s youth: toward a comprehensive assessment. Adolescent and Family Health, 4, 3-11. [ Links ]
Koul, A., Becchio, C., & Cavallo, A. (2018). PredPsych: A toolbox for predictive machine learning-based approach in experimental psychology research. Behavior research methods, 50(4), 1657-1672. https://doi.org/10.3758/s13428-017-0987-2 [ Links ]
Liaw, A., & Wiener, M. (2002). Classification and regression by randomForest. R news, 2(3), 18-22. [ Links ]
Lin, E., Lin, C. H., & Lane, H. Y. (2020). Precision psychiatry applications with pharmacogenomics: artificial intelligence and Machine Learning approaches. International Journal of Molecular Sciences, 21(3), 969. https://doi.org/10.3390/ijms21030969 [ Links ]
Liu, Y., Zhao, N., & Ma, M. (2021). The Dark Triad Traits and the Prediction of Eudaimonic Wellbeing. Frontiers in Psychology, 12, 693778. https://doi.org/10.3389/fpsyg.2021.693778 [ Links ]
Lopez, S. J., Prosser, E. C., Edwards, L. M., Magyar-Moe, J. L., Neufeld, J. E., & Rasmussen, H. N. (2002). Putting positive psychology in a multicultural context. En C. R. Snyder & S. J. Lopez (Eds.), Handbook of positive psychology (pp. 700-714). Oxford University Press. [ Links ]
Lupano Perugini, M. L., de la Iglesia, G., Castro Solano, A., & Keyes, C. L. M. (2017). The Mental Health Continuum-Short Form (MHC-SF) in the Argentinean context: confirmatory factor analysis and measurement invariance. Europe Journal of Psychology, 13, 93-108. https://doi.org/10.5964/ejop.v13i1.1163 [ Links ]
McCullough, M. E., & Snyder, C. R. (2000). Classical source of human strength: revisiting an old home and building a new one. Journal of Social and Clinical Psychology, 19, 1-10. https://doi.org/10.1521/jscp.2000.19.1.1 [ Links ]
Morales-Vives, F., De Raad, B., & Vigil-Colet, A. (2014). Psycho-lexically based virtue factors in Spain and their relation with personality traits. The Journal of General Psychology, 141(4), 297-325. https://doi.org/10.1080/00221309.2014.938719 [ Links ]
Nave, G., Minxha, J., Greenberg, D. M., Kosinski, M., Stillwell, D., & Rentfrow, J. (2018). Musical preferences predict personality: evidence from active listening and Facebook likes. Psychological Science, 29(7), 1145-1158. https://doi.org/10.1177/0956797618761659 [ Links ]
Orrù, G., Monaro, M., Conversano, C., Gemignani, A., & Sartori, G. (2020). Machine Learning in psychometrics and psychological research. Frontiers in Psychology, 10, 2970. https://doi.org/10.3389/fpsyg.2019.02970 [ Links ]
Park, G., Schwartz, H. A., Eichstaedt, J. C., Kern, M. L., Kosinski, M., Stillwell, D. J., & Seligman, M. E. (2015). Automatic personality assessment through social media language. Journal of Personality and Social Psychology, 108(6), 934. https://doi.org/10.1037/pspp0000020 [ Links ]
Park, N., Peterson, C. & Seligman, M. E. P. (2004). Strengths of character and well-being. Journal of Social and Clinical Psychology, 23(5), 603-619. https://doi.org/10.1521/jscp.23.5.603.50748 [ Links ]
Paulhus, D. L., & Williams, K. M. (2002). The dark triad of personality: narcissism, machiavellianism, and psychopathy. Journal of Research in Personality, 36, 556-563. https://doi.org/10.1016/S0092-6566(02)00505-6 [ Links ]
Peterson, C. & Seligman, M. E. P. (2004). Character strengths and virtues: A handbook and classification. Oxford University Press. [ Links ]
Ryff, C. (1989). Happiness is everything, or is it? Explorations on the meaning of psychological well-being. Journal of Personality and Social Psychology, 57, 1069-1081. https://doi.org/10.1037/0022-3514.57.6.1069 [ Links ]
Shatte, A., Hutchinson, D. M., & Teague, S. J. (2019). Machine learning in mental health: A scoping review of methods and applications. Psychological Medicine, 49(9), 1426-1448. https://doi.org/10.1017/S0033291719000151 [ Links ]
Snyder, C. R., Lopez, S. J., & Pedrotti, J. T. (2011). Positive Psychology: The Scientific and Practical Explorations of Human Strengths (2a ed.). Sage. [ Links ]
Stavraki, M., Artacho-Mata, E., Bajo, M., Diaz, D. (2023). The dark and light of human nature: Spanish adaptation of the light triad scale and its relationship with psychological well-being. Current Psychology, 42, 26979-26988 https://doi.org/10.1007/s12144-022-03732-5 [ Links ]
Tkach, C., & Lyubomirsky, S. (2006). How do people pursue happiness? Relating personality, happiness-increasing strategies, and well-being. Journal of Happiness Studies, 7(2), 183-225. https://doi.org/10.1007/s10902-005-4754-1 [ Links ]
Walker, L. J., & Pitts, R. C. (1998). Naturalistic conceptions of moral maturity. Developmental Psychology, 34(3), 403-419. https://doi.org/10.1037/0012-1649.34.3.403 [ Links ]
Yarkoni, T., & Westfall, J. (2017). Choosing prediction over explanation in psychology: Lessons from machine learning. Perspectives on Psychological Science, 12(6), 1100-1122. https://doi.org/10.1177/1745691617693393 [ Links ]
Zou, H., & Hastie, T. (2005). Regularization and variable selection via the elastic net. Journal of the Royal Statistical Society: Series B (statistical methodology), 67(2), 301-320. https://doi.org/10.1111/j.1467-9868.2005.00503.x [ Links ]
Funding: This work was carried out through UBACyT grant 20020190100045BA "Psychological profile of the Internet and social network user. Analysis of positive and negative personality characteristics from a psycholexical approach and mediating psychological variables"
How to cite: Castro Solano, A., Lupano Perugini, M. L., Caporiccio Trillo, M. A., & Cosentino, A. C. (2024). Validation of a model of positive and negative personality traits as predictors of psychological well-being using machine learning algorithms. Ciencias Psicológicas, 18(1), e-3286. https://doi.org/10.22235/cp.v18i1.3286
Authors’ contribution (CRediT Taxonomy): 1. Conceptualization; 2. Data curation; 3. Formal Analysis; 4. Funding acquisition; 5. Investigation; 6. Methodology; 7. Project administration; 8. Resources; 9. Software; 10. Supervision; 11. Validation; 12. Visualization; 13. Writing: original draft; 14. Writing: review & editing. A. C. S. has contributed in 1,3,4,6,7,9,10,13,14; M. L. L. P. in 3,6,12,13,14; M. A. C. T. in 13,14; A. C. C. in 1,8,13,14
Received: March 10, 2023; Accepted: April 30, 2024